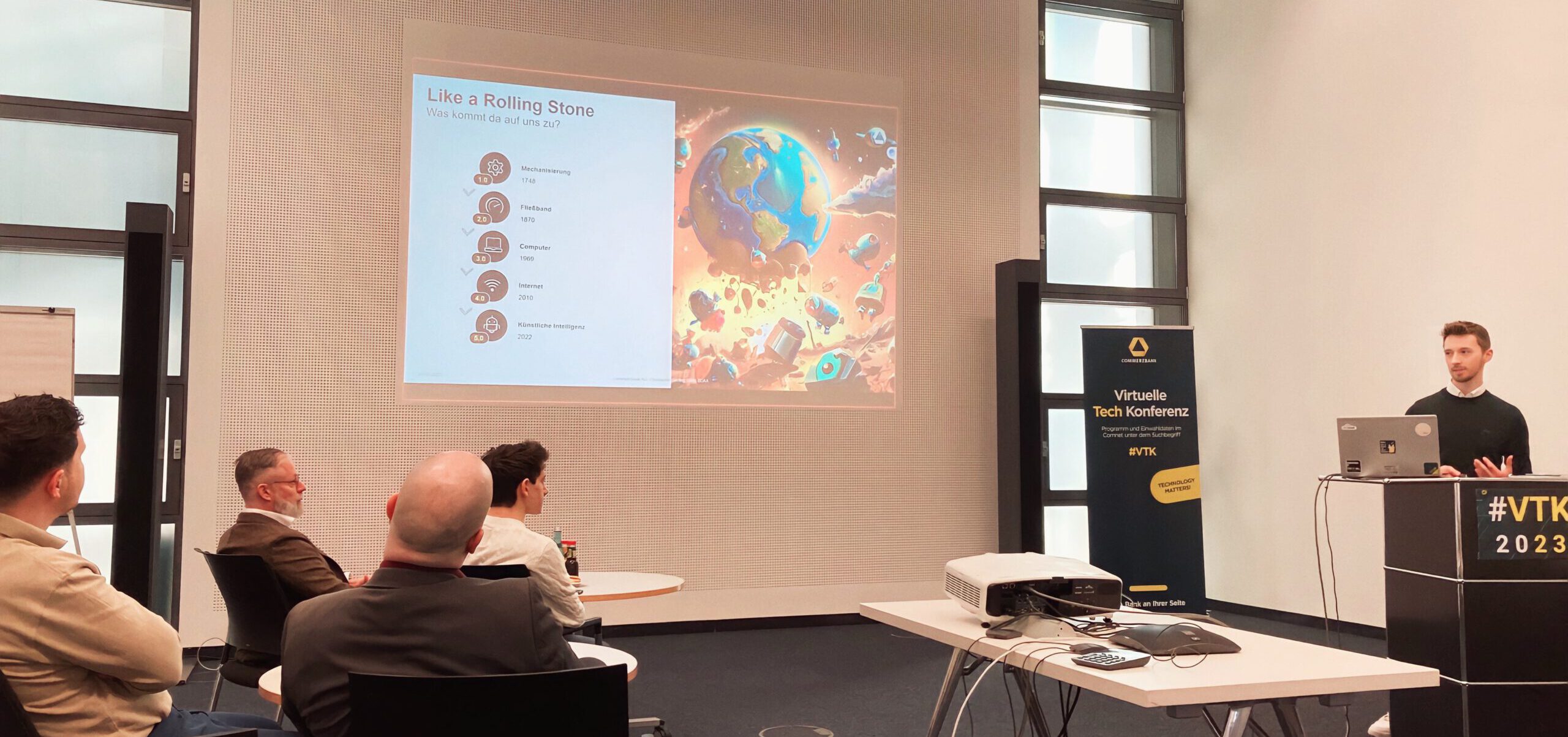
In the ever-evolving world of finance, staying ahead of the curve is a necessity for banks and financial institutions. Recently, I had the privilege of delivering a presentation to over 300 professionals from the banking industry, shedding light on how artificial intelligence (AI) will revolutionize the future of banking. As a data scientist specializing in the banking sector, my goal was to explore the potential of AI in transforming the industry and the challenges and opportunities that lie ahead.
The 5th Industrial Revolution: Transforming the Banking Sector in the Age of AI and Automation
The 5th industrial revolution, often referred to as the Intelligence Revolution or Industry 5.0, is characterized by the fusion of cutting-edge technologies, such as artificial intelligence (AI), automation, the Internet of Things (IoT), blockchain, and biotechnology. This revolution is driving unprecedented change, blurring the lines between the digital, physical, and biological realms, and profoundly affecting various industries, including banking.
In this new era, the banking sector is poised for a paradigm shift, as traditional operations and business models are disrupted by transformative technologies. To remain competitive and relevant in the rapidly changing financial world, banks must adapt to the new landscape forged by the 5th industrial revolution. This involves embracing advanced technologies, rethinking traditional business models, fostering collaboration, and prioritizing sustainability and social impact. By proactively responding to the opportunities and challenges presented by the 5th industrial revolution, banks can secure their position in the future of finance and continue to drive growth and innovation.
AI versus Machine Learning
AI is a broad concept encompassing the development of machines capable of carrying out tasks that would typically require human intelligence. Machine learning (ML), on the other hand, is a subset of AI, focusing on the development of algorithms that enable machines to learn and improve from experience. While AI is the overarching concept, ML is the driving force behind the practical applications of AI in various industries, including banking.
From a regulatory perspective, even complex data analyses are considered AI, as they involve the use of advanced algorithms to process and make sense of large amounts of data. This classification underscores the importance of understanding the relationship between AI and machine learning, as it has significant implications for banks and financial institutions.
As regulatory bodies across the globe are increasingly recognizing the potential impact of AI on the financial sector, they are developing guidelines and frameworks to ensure its responsible and ethical implementation. For instance, the European Union has proposed regulations that focus on transparency, accountability, and data protection in AI systems, while the United States has established guidelines for the use of AI in the financial services sector.
In this context, banks and financial institutions must be aware of the evolving regulatory landscape and adapt their AI and machine learning strategies accordingly. This includes ensuring compliance with existing regulations, actively engaging with regulators to shape future policies, and adopting best practices for responsible AI implementation.
Applications of AI in Banking: Exploring the Possibilities with Real-World Examples
AI has the potential to transform the banking industry in numerous ways, making financial services more efficient, cost-effective, and customer-centric. In this section, we will explore the various applications of AI in banking, illustrating its impact with real-world examples.
Sales Revenue Growth
AI-powered customer analytics and personalization can help banks create highly targeted marketing campaigns that cater to individual needs and preferences. For example, using AI to analyze customer data, banks can identify patterns in spending habits and recommend relevant products or services, such as loans, investment options, or insurance policies. This personalized approach not only improves customer satisfaction but also increases cross-selling and up-selling opportunities, ultimately driving sales revenue growth.
AI can also play a significant role in enhancing customer experience by offering personalized, timely, and relevant services. From virtual assistants to AI-driven credit decisioning, the use of AI in banking can significantly improve customer satisfaction and loyalty, which then can increase sales activities.
Cost Reduction and Automation
By automating manual and repetitive tasks, AI can significantly streamline banking operations, increasing efficiency and reducing operational costs. For instance, AI-driven chatbots can handle a vast majority of customer inquiries, freeing up human employees to focus on more complex tasks and strategic initiatives.
Fraud Detection and Prevention
AI-powered algorithms can analyze vast amounts of data in real-time, identifying patterns and anomalies that may indicate fraudulent activities. This enables banks to detect and prevent fraud more effectively and efficiently than traditional methods.
Risk Calculation
AI can process complex data sets and uncover hidden patterns, improving risk assessment, credit scoring, and portfolio management. By leveraging AI for these tasks, banks can make more informed decisions and minimize potential risks.
Balancing Opportunities and Threats: Navigating the Ethical Landscape of AI in Banking
The integration of artificial intelligence (AI) into the banking sector brings forth a wealth of opportunities, while simultaneously raising concerns about potential threats and ethical challenges. To harness the full potential of AI and maintain a competitive edge in the fast-paced world of finance, banks must balance the opportunities and threats, adopting a responsible and ethical approach to AI implementation.
AI presents numerous opportunities for banks to enhance customer experiences, streamline operations, and drive innovation. By leveraging AI, banks can improve decision-making, identify new business opportunities, and maintain a competitive edge in the rapidly evolving financial landscape.
However, as AI becomes increasingly integrated into banking, ethical concerns must be addressed to maintain trust and protect human interests. Some of the primary threats and ethical challenges associated with AI in banking include:
- Data privacy: The use of AI-driven analytics raises concerns about customer data privacy and the potential for misuse or unauthorized access to sensitive information.
- Discrimination: AI algorithms may inadvertently perpetuate biases present in the data they analyze, leading to unfair treatment or discrimination against certain customer segments.
- Job loss: The automation of tasks through AI may result in job displacement, particularly for roles involving manual and repetitive tasks.
- Algorithmic transparency: The use of complex AI algorithms in decision-making processes may lack transparency, making it difficult for customers to understand how decisions are being made.
To balance these opportunities and threats, banks must adopt a proactive and responsible approach to AI implementation, focusing on the following strategies:
- Establish ethical guidelines: Banks should develop ethical guidelines and frameworks for AI implementation, ensuring that AI systems are designed, deployed, and monitored responsibly.
- Promote transparency: Banks should strive for algorithmic transparency, clearly communicating how AI-driven decisions are made and ensuring customers have the right to contest or inquire about such decisions.
- Address biases: Banks should actively work to identify and mitigate biases in AI algorithms, ensuring fair treatment for all customers.
- Encourage re-skilling and up-skilling: Banks should invest in employee training and development programs, enabling workers to adapt to the changing landscape and secure new roles within the organization.
In conclusion, to harness the power of AI in banking while addressing the associated threats and ethical concerns, banks must adopt a balanced approach. By focusing on responsible AI implementation and promoting transparency, banks can capitalize on the opportunities presented by AI, driving innovation and maintaining a competitive edge in the rapidly evolving financial world.